What Is Design for Reliability (DFR)? Overview & Applications
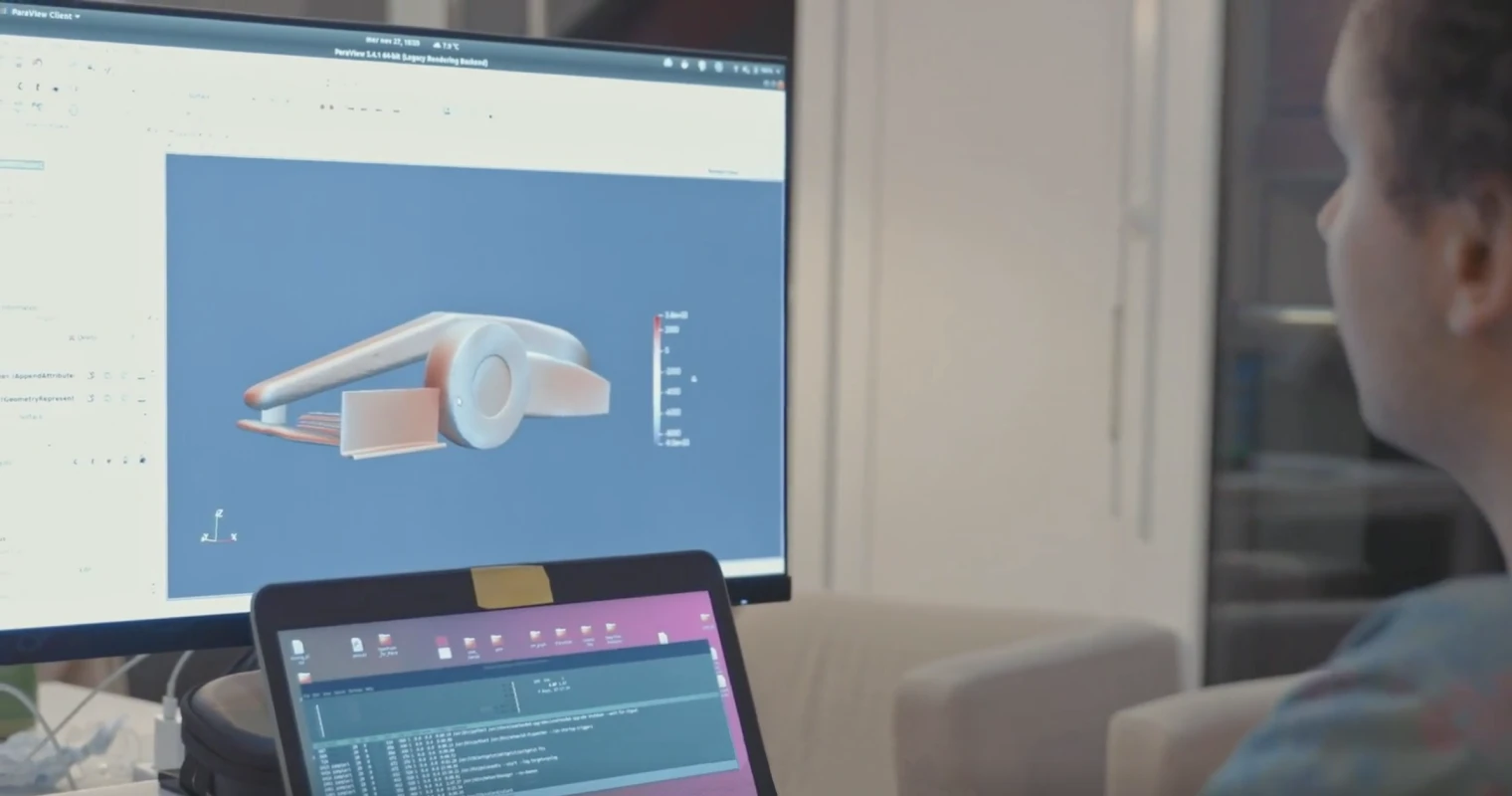
Reliability engineering predicts and prevents product failure through careful analysis, design, testing, and continuous improvement. Design for reliability involves assessing the probability of successful performance over time, addressing wear-out mechanisms, and ensuring sustained product functionality under specified conditions.
In this article, we will see how reliability engineers enhance product dependability and minimize the risk of unexpected failures — a topic of huge interest to engineers and “for the rest of us”!
Dramatic failures can stem from a lack of robust reliability engineering behind a product.
This is why product reliability is a critical factor in customer satisfaction and brand reputation. Companies can enhance customer trust and establish a more positive brand image by implementing reliability engineering.
A key focus will be on predicting failures before they occur.
Predicting Reliability
Predicting reliability is a fundamental component of Design for Reliability (DFR).
“Prediction” means estimating the likelihood of a failure during the product life cycle under specific conditions. Thus, in design for reliability (DFR), we focus on failure analysis before it occurs. Reliability engineering experts identify potential failures and recommend appropriate solutions with a wide range of reliability analysis tools, which we will describe.
Design for reliability aims to ensure a product or system’s reliability throughout the product life cycle, focusing on the design stage. Potential failure modes are identified through suitable design, testing, and later monitoring.
Given the importance of product reliability and the continuous growth of statistical analysis methods, there is a growing emphasis on design for reliability within product development teams. Reliability engineers operate preemptively in the early stages, and their recommendations are valid throughout the manufacturing and assembly processes that occur in the product life cycle downstream of design.
Lessons Learnt
One key lesson from cases such as the NASA Challenger disaster [1] is the importance of design for reliability. The O-ring seals on the rocket boosters were a critical component of the Space Shuttle system, and their failure led to the loss of the vehicle and its crew.
This failure could have been avoided if adequate full reliability analysis and engineering tools had been used during the boosters’ design and development.
To recall a recurring concept in this article, reliability prediction analyzes a product or system to identify failures and their causes and develops strategies to mitigate or eliminate them.
This process involves analytical techniques, such as stress analysis with finite elements (FEA) and Failure Mode and Effects Analysis (FMEA), testing, and validation.

In the case of the failure reporting the booster O-ring seals, a prediction could have identified the seals' susceptibility to failure in cold weather conditions, strategies could have been developed to mitigate that risk, and alternative seal designs or materials could have been explored, or launch criteria could have been established to prevent launches in cold weather conditions.
However, without reliability analysis, these steps were not taken, and as a result, the Challenger disaster occurred with tragic consequences.
Today, reliability prediction is a standard part of most complex engineering systems’ design and development process, extending beyond the aerospace industry to the automotive design and manufacturing process and any other manufacturing process or critical energy systems like nuclear plants.
The Space Shuttle Challenger disaster is a dramatic example of the consequences that can arise when a product or system is launched without adequate reliability predictions.
General Considerations on Design for Reliability
Design for reliability is a systematic approach to product development that considers reliability at every stage of the product life cycle, from early concept to manufacturing process, before deployment to users.
It involves identifying potential failure modes and their causes, developing strategies to mitigate those failures, and validating the effectiveness of those strategies within the product life cycle.
Design for reliability also involves considering factors such as manufacturing processes, materials selection, and operational conditions. All of these can have an impact on product reliability.
In contrast, designing without reliability prediction can lead to various adverse outcomes. In such a process, we can identify:
Increased likelihood of product failure. Without adequate reliability prediction, products may be more prone to failure, resulting in increased costs, lost revenue, and reputation damage.
Safety risks. Failure of critical components can pose safety risks to users, leading to injury or even death.
Recalls and litigation. Product failure can result in recalls and litigation, which can be costly and damage a company’s reputation. The field of company recalls is central to reliability engineering. This is why:
As a first case, imagine a company that manufactures and sells electronic components. Due to a design flaw due to poor usage of reliability tools in the design stage, some of these devices pose a fire hazard. The company identifies the issue and initiates a recall, notifying affected customers to return or repair their devices. Such a process prevents potential accidents, protects consumers, and fulfills regulatory obligations. While the recall may incur costs (e.g., logistics, replacement parts) and temporarily damage the product’s reputation, it prevents injuries, lawsuits, and further long-lasting reputation loss.
In the case of automotive defects, an automobile manufacturer discovers a defect in a critical safety component (e.g., airbags, brakes) that was not identified in the product development process. The manufacturer issues a recall, urging vehicle owners to visit authorized service centers for repairs or replacements. This prevents accidents and ensures compliance with safety standards. The recall process involved expenses; however, at least it mitigated potential lawsuits and injuries, even with temporary negative publicity and the reputation of a reliable product.
In a Food and Beverage recall, a food company identified contamination in a batch of its products (e.g., bacterial contamination in packaged salads) that was not foreseen before with statistical methods. The company immediately recalled the affected products from store shelves, notified consumers, and provided refunds or replacements. This safeguarded public health and maintained consumer trust. While the recall prevented illnesses and lawsuits, it damaged the brand’s image.
Missed opportunities. Failing to consider reliability early in the design process can lead to missed opportunities for cost savings and performance improvements. One example are innovative reliability tools like AI in design process iterations when deployed within the product life cycle.
They have reduced customer satisfaction. Unreliable or prematurely failed products can reduce customer satisfaction, negatively impacting a company’s bottom line.

In contrast to the Challenger disaster, many examples of companies have successfully implemented DFR and reaped benefits.
For example, Toyota is known for its focus on quality and reliability in its automotive products. This has helped the company build a strong reputation and customer satisfaction, which has translated into increased market share and revenue.
Another example is the medical device industry, which has recently seen significant improvements in reliability and safety as a result of increased focus on design for reliability. The implementation of rigorous tests and prediction processes has led to developing safer medical devices that have improved patient outcomes and reduced risk of adverse events.
In conclusion, past disasters serve as a reminder of the importance of designing for reliability. Failing to consider this early in the design process can lead to severe consequences, including product failure, safety risks, recalls, missed opportunities, and reduced customer satisfaction.
Let us close this section on a positive note.
Implementing design for reliability can help companies build a strong reputation for quality and reliability, which can translate into increased market share and revenue. As such, designing for reliability should be an integral part of the design process for any complex engineering system.
The Importance of Product Reliability in Design
Reliability of design is crucial for ensuring customer satisfaction, and the return for manufacturers is a reduction in warranty costs and protecting a company’s reputation.
Performance is essential, but a reliable product enhances customer trust and loyalty and helps to establish a positive brand image. Additionally, designing for reliability can lead to cost savings for the producers by reducing customer warranty claims or “class actions.”
Product quality and product reliability are two critical factors that determine the overall value of a product. While these terms are often used interchangeably, quality and reliability refer to different aspects. While product quality and reliability are closely related, they are not the same.
How do we model complex systems for reliability?
Reliability block diagrams are a standard tool used in reliability engineering. They break down a system into individual components and calculate the system’s overall reliability based on each component’s reliability and interdependencies.
To design for reliability, product development teams should identify KPIs such as reliability risks and requirements. The requirements are the product’s expected performance standards, including lifespan, performance under different conditions, and failure rate.
The product development team should define these requirements as early as possible in the design process to ensure they are incorporated into the design.
The Design for Reliability Process
The design for reliability process involves several steps, including identifying potential failures, addressing them through appropriate design solutions, and testing and validating the reliability tools and design. The following are the minimum steps in the reliability assessment process:
Identify. The first step in the Design for Reliability process is identifying potential failures. This involves identifying the different ways the product can fail, such as through crash test simulation or electrical or thermal stress simulations.
Design. Once potential failures have been identified, the reliability engineer’s next step is to design solutions. These can include selecting appropriate materials, adding redundancy, implementing error-checking, or incorporating fail-safe mechanisms.

Analyze. After designing solutions, product development uses reliability engineering tools such as Failure Mode and Effects Analysis (FMEA) or Fault Tree Analysis to identify potential failure points and evaluate the impact of failures on reliability.
Fault Tree Analysis originated in the 1960s during the evaluation of the Minuteman I Intercontinental Ballistic Missile (ICBM) Launch Control System.
FTA represents the failure analysis through a graphical model called a fault tree. This tree visually depicts the logical relationships between events and their impact on the top event (the undesired state).
Test. The design must be tested under different environmental conditions to meet the reliability requirements. Testing can include environmental testing, accelerated life testing, and stress testing.
While laboratory/physical testing is an essential step in designing for reliability, it is important to note that modern tools like computer-aided engineering (CAE) and artificial intelligence (AI) can also play a significant role in the reliability design process. These tools can help designers and engineers identify potential reliability issues before beginning physical testing.
CAE software allows designers to simulate a product’s behavior under various conditions, such as temperature, loads, or vibration, to simulate mechanical shock in electronic components. Simulation can reveal potential weaknesses in the design that could lead to reliability issues down the line. By identifying these issues early on, designers can make changes to the design in the CAD stage to improve its reliability before physical testing begins.
AI prediction can also reveal a product’s reliability based on similar product or component data. By analyzing data from various sources, AI algorithms can identify patterns and predict potential failures in a new product.
An example can be the simulation of a PCB drop test [2] for which consolidated literature exists but now, thanks to AI in almost real-time and other types of mechanical shock prediction. This information can be used to make design changes or prioritize certain areas of the product for testing.
Once testing is complete, it is validated to meet the reliability requirements. This involves comparing the product’s performance against the expected performance defined in the reliability requirements.
Finally, the product must be monitored throughout its lifecycle to ensure it meets the reliability requirements. This can involve monitoring the product’s performance in the field, collecting customer feedback, and conducting periodic reliability testing.
In conclusion, designing for reliability goals is a critical aspect of product development that helps to ensure consistent performance over time.
It involves identifying potential failures, addressing them through appropriate design solutions, and testing and validating the design to meet reliability requirements.
Following the Design for Reliability process, product development teams can create products that meet customer expectations, reduce warranty costs, and protect a company’s reputation.
Design for Reliability Success Story - How Toyota Became a Giant
The Toyota Corolla is a legendary car for its longevity. With few significant problems and low annual maintenance costs, it is not unusual for dedicated owners to hit 500,000 km or higher. So, let us analyze the success story behind the Toyota Corolla.
First, Toyota prioritized reliability and longevity during the Corolla’s design and manufacturing, so design for reliability was part of the car’s DNA. The Corolla’s design emphasized simplicity and practicality, focusing on essential features and functionality.
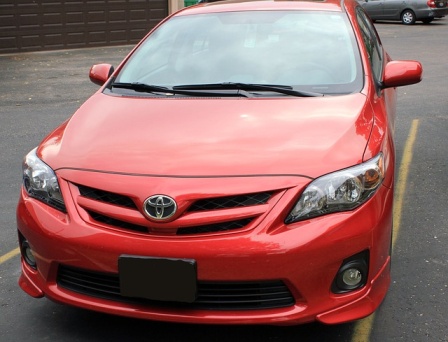
Thus, Corolla consistently delivered on Toyota’s promise of trouble-free ownership.
Moreover, the Corolla’s relatively high fuel efficiency was a “winner” during the oil crisis of the 1970s.
The enduring impact of the Corolla’s careful design for reliability was record sales (over 50M units sold globally). Meeting customer expectations created loyalty with repeat buyers, and in the end, the Corolla became a sort of “pop icon” [3].
Design Reliability Applications and Artificial Intelligence
DFR has traditionally been a process that involves identifying potential failure modes, designing solutions, analyzing the design, testing the design, validating the design, and monitoring the product’s performance throughout its lifecycle. AI has the potential to revolutionize each of these steps, providing new opportunities for improving product reliability and reducing life cycle costs.
Life cycle costs encompass various expenses associated with a product or system throughout its life span. These include the initial purchase price and ongoing expenses such as maintenance, repairs, spare parts, and downtime. Reliability analysis supported by AI can reduce product costs while shortening the time to market.
Identifying Potential Failure Modes
AI has shown significant promise as more reliability data are available to feed statistical analysis tools to identify potential failure modes. Machine learning algorithms can analyze bunches of data to identify patterns and anomalies that might indicate potential failure modes.
For instance, AI algorithms have been used to analyze flight data to identify potential safety issues before they become critical: algorithms can detect trends and patterns that might indicate a failure mode and alert engineers to the issue before it becomes a problem.
Design for Reliability
Designing solutions is another area where AI can significantly impact the industry. Generative design, a technique that uses algorithms to create designs based on input criteria, has shown great promise in improving the design process.
Generative design algorithms can quickly generate thousands of potential designs by inputting criteria such as weight and material properties. These designs can then be evaluated using simulation tools to determine their performance and reliability.
However, trusting any software 100% can be a mistake.
Reliability testing ensures the software meets its intended purpose, maintains consistency, and delivers accurate results. Reliability testing contributes to a more robust and dependable software system by identifying faults and providing quality assurance.
AI in Design for Reliability
The application of AI in DFR can revolutionize the product development system reliability analysis process. By leveraging AI, reliability engineering experts can more accurately identify failure mechanisms and design more reliable and robust products. AI can also help identify failure mechanisms that may not be apparent through traditional reliability analysis techniques.
Failure Mechanisms in Design for Reliability
Let us first introduce the concept of identification and mitigation of failure mechanisms central to design for reliability.
Failure mechanisms are the physical processes that result in product failures, such as fatigue, corrosion, wear, and fracture. Identifying failure mechanisms is critical for designing reliability via mitigation strategies to prevent or delay failure.
Several reliability analysis tools can leverage experimental testing, simulation, and field data analysis to identify failures.

An example of field failure data involves collecting data on product failures in the field and analyzing that data to identify failures. This approach can help identify failure mechanisms that may not have been anticipated during the design stage.
The following section will discuss using failure data for a new set of reliability analysis tools based on AI.
Machine Learning for Failure Mechanism Identification
Machine learning (ML) is an AI branch presenting exciting opportunities for identifying failure mechanisms and implementing effective mitigation strategies. By analyzing extensive data from complex systems, ML algorithms can uncover patterns that traditional methods might miss since they can detect unforeseen failures during the design phase. This allows reliability engineering teams to enhance mitigation strategies before they occur in a genuine design for reliability approach.
A significant advantage of ML in reliability design lies in its ability to handle large datasets from diverse sources, including sensor data, past simulations, and real-world observations. Reliability engineers can leverage ML with its statistical analysis methods throughout the product life cycle, especially in the reliability preemptive phases.
Case Study: Machine Learning for Failure Mechanism Identification
One example of the application of ML for failure identification is in the aerospace industry. Failure of critical components can have catastrophic consequences, making early prognostics of such a process a top priority for aerospace engineers and research centers. To address this challenge, researchers at NASA have developed an ML-based approach for identifying failure mechanisms in aerospace systems. The strategy involves training ML algorithms on large volumes of data from various sources, including sensor, simulation, and field data.
Conclusions
We have seen how design for reliability is a critical aspect of product development that aims to minimize the risk of failure and ensure long-term product performance.
One key challenge in reliability design is identifying potential failure mechanisms rather than post hoc failure reporting. Thus, products must be designed to mitigate failures before they occur.
What are the new opportunities for identifying failure mechanisms that may not have been anticipated during the design phase, enabling engineers to design more effective mitigation strategies?
Failure mechanisms are at the heart of DFR/Design for Reliability. Incorporating AI and machine learning into the design for reliability helps to accurately identify failure mechanisms and design products that can withstand the demands of real-world use.
References
[2] for instance, https://us.toyotaownersclub.com/forums/forum/4-corolla-club/
[3] https://www.circuitinsight.com/pdf/predicting_lifetime_pcb_ipc.pdf