Simulation Crash Testing: Enhancing Automotive Design
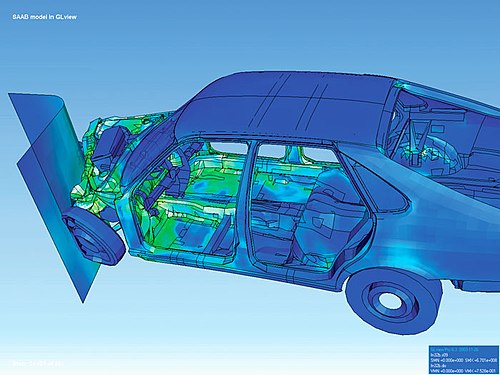
This article is dedicated to all drivers and passengers... although we hope you will always only see dummies involved!
Crash simulations help to improve safety, reduce development costs, and accelerate the automotive design process with respect to traditional prototype-and-test procedures.
Crash simulations continue to evolve. They are now incorporating AI technology that feeds on generated simulations. This recent evolution could take place because in the last years, a large amount of simulation has been carried out and stored. And, even now while you are reading the article, some automotive engineer has probably just completed her standard professional simulation and enriched the company database. Hence, the AI potential has grown with new inputs to learn from to hone its modeling capability.
Within the automotive digital thread, the AI solution, as complementary to the consolidated FEA approach, promises to revolutionize further how cars are designed and tested for safety while reducing development costs, giving designers a new predictive capability without having to learn physical modeling.
A Little Bit of History From Crash Tests to the Computer Era
The traditional robust process was not based on computers and models but on experimental testing methods, where dummies with sensors were placed on the driver (and passenger) seat. The most known type of test is a car impinging on a solid (cement) wall with a frontal impingement. Such tests led to influence the market with models that were taken out of the market because of insufficient safety ratings.
Real crash tests were later helped by computer-aided engineering, i.e. car simulation performed on dedicated computers to fine-tune the understanding of crashworthiness and better reproduce it, especially in terms of design changes. Imagine replacing a physical part and running a new test Vs changing CAD files and launching a new simulation. The finite element model provides faster results and helps accelerate development with feedback stored company datasets based on well-known physical modeling, i.e. with a robust process behind it.
Later, many more tests were developed, such as side-impact, side pole-impact, or roll-over, and the finite element method helped to tackle a large number of design changes to help engineers determine the best car models with calculations.

New Technologies for Crash Simulation
Designers can now wonder if the development from physical to virtual crash has any further steps in helping engineers simulate mechanical processes and implement new materials for the automotive industry.
The answer is that AI can help predict a crash event - when fed with quality datasets! Data-driven AI prediction does not "solve" a crash event with a finite element solution but rather uses all the cumulated data from the past (dataset) to evaluate any crashworthiness case with unprecedented speed (0.1 - 1 sec). This is very helpful for designers to discriminate geometric or material features and identify the best solution. We will give two case study examples of such a data-driven process to complement testing and simulation software (how AI can accept and incorporate any source with the data fusion concept).
What Is Crash Simulation and Car Crash Simulation?
A crash simulation is a computer-based technique to model and analyze how vehicles and their components behave during collisions. These simulations employ advanced software to replicate real-world crash scenarios, providing engineers with a virtual testing environment to assess the safety and performance of vehicle designs.
We will focus on the automotive sector and car crashes.
When conducted using advanced FEA (finite element analysis) and other simulation techniques, a virtual car crash simulation can achieve high realism in modeling structural deformations, vehicle behavior, and occupant dynamics during crashworthiness testing. These simulations can provide visual insights and detailed numerical reports into vehicle integrity, safety, and performance.
Crash simulations offer a range of benefits to the automotive industry. They enable faster and more cost-effective testing, facilitate design improvements, and enhance safety. Simulations can help reduce the need for physical crash tests, saving time and resources. All major OEMs are equipped with FEA teams using dedicated software and hardware.

The success of crash simulations hinges on specialized software and tools. These applications allow engineers to create realistic vehicle models, set crash scenarios, and analyze results. Leading software packages such as ANSYS LS-DYNA, Altair Radioss, ESI Pam-Crash or Simulia Abaqus/Explicit have the support of high-performance computing (HPC) hardware. With HPC, the simulation based on the finite element method is performed in a reduced time, for instance, one-tenth or less of the time required to run on a CAD workstation.
In practice, automotive engineers use combinations of physical crash tests and simulations to design and test vehicles for safety. Simulations are valuable for initial design iterations, sensitivity analysis, and efficient exploration of safety ratings in various scenarios. However, physical crash tests remain essential for final certification, and understanding of how real-world variable impact safety performance.
Data Collection and Model Creation
Engineers collect data on vehicle components and create accurate 3D models, ensuring that simulation is based on real-world parameters.
The challenges here are twofold.
Firstly, data collection can be time-consuming and costly, requiring precise measurements of each component's geometry and material properties.
Secondly, creating detailed 3D models demands CAD (Computer-Aided Design) software expertise to represent complex vehicle structures accurately.
Setting Up Crash Scenarios
Engineers define crash scenarios, specifying impact conditions, angles, and speeds to replicate various collision scenarios.
Regulatory tests from the US and EU authorities, namely the National Highway Traffic Safety Administration (NHTSA) in the United States and the European New Car Assessment Programme (Euro NCAP) in Europe, mandate specific crash test scenarios. These crash test scenarios ensure that cars meet safety standardized conditions.
The challenges here are adapting simulation to diverse regulatory requirements and accounting for real-world variability.
Running Simulation Software to Simulate Car Crashes
Simulation is executed using specialized software, modeling vehicle elements during a crash in a virtual environment.
Finite element analysis commercial software is used to simulate complex deformations in the vehicle structure, starting from a car model in CAD format. To model real crash tests, today's crash simulations include virtual models of crash test dummies and passive safety devices such as seat belts, airbags, or shock-absorbing dashboards..
Analyzing Results
Engineers analyze simulation results to assess structural integrity, occupant safety, and overall performance.
Important results are the deformations (for example, steering wheel intrusions) of the occupant space (driver, passengers) and the decelerations (for example, head acceleration) felt by them, which must fall below threshold values fixed in legal car safety regulations.
Simulation Challenges and Limitations
Despite their advantages, a crash simulation also comes with challenges and limitations. Ensuring that a simulation accurately represents real-world events and validating simulation results will remain ongoing challenges. Validation involves comparing simulation outcomes with physical crash test data to verify the accuracy of the virtual models.
Also, high-performance computing infrastructure and software licenses can be costly, limiting access for some users and some organizations. Simulating complex crash scenarios often demands significant computational resources. This can be a barrier for smaller companies or research institutions with limited budgets.
On-demand cloud solutions have been recently offered to alleviate the need to build and maintain an internal IT structure.
Deep Learning Technology: Fast Car Crash Simulations without Fast Solver?
A case study showcases how car crash simulations are applied in real-world automotive design, showcasing the technology's practical impact.
Neural Concept's innovative use of AI and machine learning technology with a real-time predictive model demonstrates how these technologies can enhance the accuracy and efficiency of a crash simulation, ultimately leading to safer vehicles and reduced car development costs. This case study serves as a testament to the transformative potential of crash simulation in the automotive industry.
Case Study 1: Improving Car Crashbox Performance by 10%
To illustrate the application of deep learning in crashbox design, a frontal head-on impingement scenario for the Urban Modular Vehicle is considered. The initial design of the aluminum alloy crashbox and several design variables were evaluated.
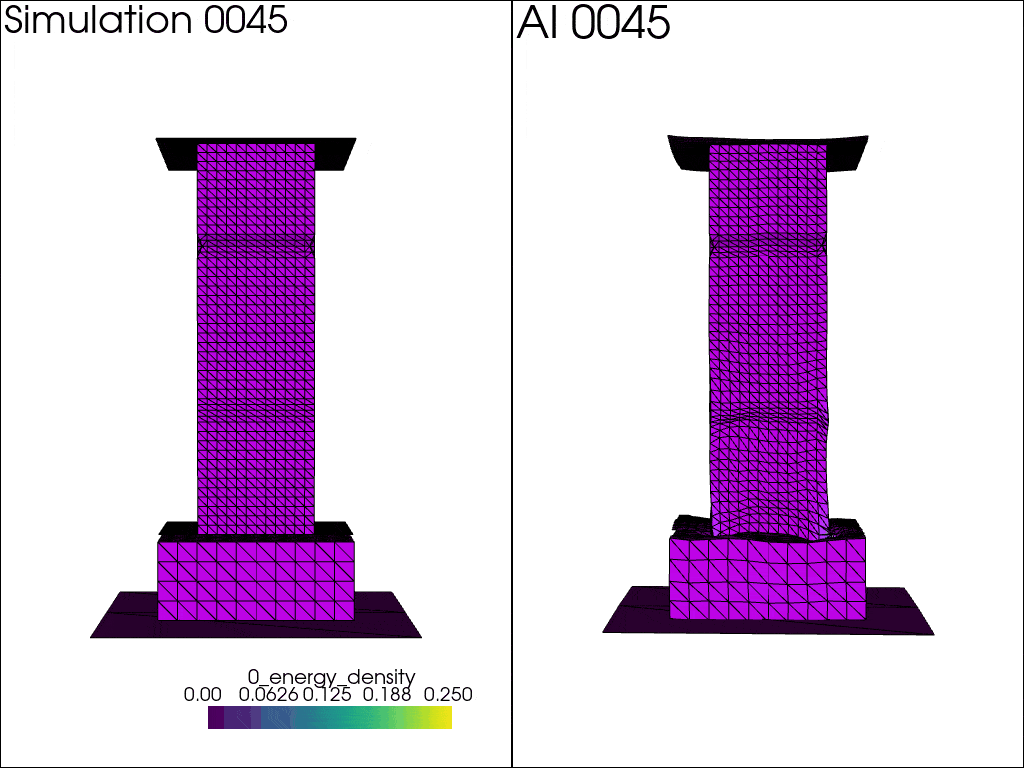
The aim was to generate an optimal lightweight design within constraints on the geometry while maximizing the Specific Energy Absorption (SEA).
The surrogate AI model trained using the dataset was compared to finite elements simulation outputs, demonstrating its accuracy and reliability in prediction. Trained from various parametric crashbox designs, the model could predict the outcome of new (never seen before) designs with high accuracy on both the scalar and field quantities.
Case Study 2: Side Pole Impact for Car Battery Encasing
The outcome of a successful implementation of a crashworthiness prediction tool was threefold:
- to help the company capitalize on its own accumulated knowledge and experience in terms of stored dataset
- to construct a design verification tool usable by the company's design engineers
- to assess crashworthiness in 3D with the same look and feel as finite elements analysis

The AI-driven design process described here is revolutionary. A designer without specific crash simulation skills can obtain displacement and maximum force in just a few seconds. This feedback time hugely improves the previous lead time of several hours that designers had to wait between CAD design conception and CAE (FEA) verification. This innovative approach fulfills the objective of simulation-driven design with faster iterations and more variables explored simultaneously. Moreover, further development, such as algorithm-driven design. This involves optimization algorithms driving design changes, which is expected for the future of "virtual crash".
Future Trends in Vehicle Crash Simulation Technology
The future of car crash simulations holds several exciting prospects, including the following three.
Industry and Regulatory Impacts
As simulations become more accurate and reliable, they will likely play a more prominent role in meeting industry safety standards and regulatory requirements.
Development in Simulation Technology
Evolving software and hardware technologies will continue to enhance the accuracy and efficiency of crash simulations.
AI and Machine Learning Integration Development
Artificial intelligence and machine learning integration will enable more sophisticated predictive modeling and data analysis, with unprecedented response speed and enhanced accuracy as datasets grow and are incorporated in the learning process.