Revolutionizing Generative AI Product Design for Innovative Solutions

Generative AI in Product Design: Benefits and Challenges
Traditional design relied heavily on product designers’ creativity and intuition. Does anyone remember those armies of engineers bent over their drafting machines? However, as challenges grew, the product design methods we relied on had to evolve.

What is the most evolutionary product design methodology we have today? Generative Design supported by AI is the answer.
Generative AI is a new approach that leverages data-driven software tools deemed impossible even 20 years ago.
Rather than manual iterations, AI algorithms are the basis of design iterations for two primary reasons.
(1) Response Time. AI has a response time that is orders of magnitude faster than any human; its data-driven technology can generate optimized design options rapidly. Also, AI can outperform most traditional software, such as physical modeling (CAE), in terms of speed of exploration of design spaces.
(2) Adherence to Data. AI relies on training data, which minimizes human bias—human intuition can be subjective. AI continuously learns from datasets and adapts to new information, providing consistent and data-aligned outputs. Thus, AI spawns designs driven purely by measurable parameters. Thus, unprecedented speed is exploited to allow users to explore the “design space” beyond human-conceived solutions, and algorithms identify unexpected, counterintuitive designs.
This is, in short, generative AI in product design.
Now, will generative AI technology replace engineers?
AI does not understand what it is doing, even if we think understanding natural language and following textual prompts are signs of intelligence. A generative AI tool leverages complex algorithms to learn how to respond to human input. Generative AI-powered design tools enable teams to convert text prompts into high-quality UI designs rapidly.
Generative AI uses data that leverages users’ experience and learns to associate user interaction patterns with probable outcomes. Thus, AI adoption will alleviate repetitive tasks on “modified input—requested output” and enhance human creativity by freeing engineers to focus on strategic and innovative aspects of their work rather than routine processes focused on incremental changes.

Generative AI product design is an innovative process that generates, tests, and iterates options based on set constraints and objectives, streamlining workflows with data-driven decision-making for product designers.
Generative AI technology requires a dataset of relevant design examples as a starting point. Examples include historical performance data, engineering outputs from testing or simulation, material specifications, and engineering constraints provided by product designers. The model learns patterns in the data, enabling users to produce designs within the specified parameters while considering usability and durability factors.
Neural Concept has 3D Deep Learning capabilities, uniquely positioned to harness generative AI for design optimization.
What is Generative AI in Product Design?
Generative AI in product design uses advanced algorithms, particularly deep learning, to automatically generate multiple design variations based on specific performance criteria. In traditional design workflows, users manually adjust parameters and run simulations. Generative AI, in contrast, uses predictive models trained on vast datasets to explore design spaces autonomously.
Current Status
In 2023, the world “discovered” generative AI. 2024 will be recorded as the year organizations began using this technology and deriving business value. In the latest McKinsey Global Survey on AI, 65% of respondents answered their organizations regularly use generative AI. This is a nearly 100% increase over less than one year.
Deep Learning Technology
Deep learning algorithms, especially neural networks, are trained to understand complex relationships between design inputs and outputs, i.e., key performance metrics relevant to product development and specific to the industry.
Once trained, models generate optimized designs for product designers, predicting performance metrics as the above.
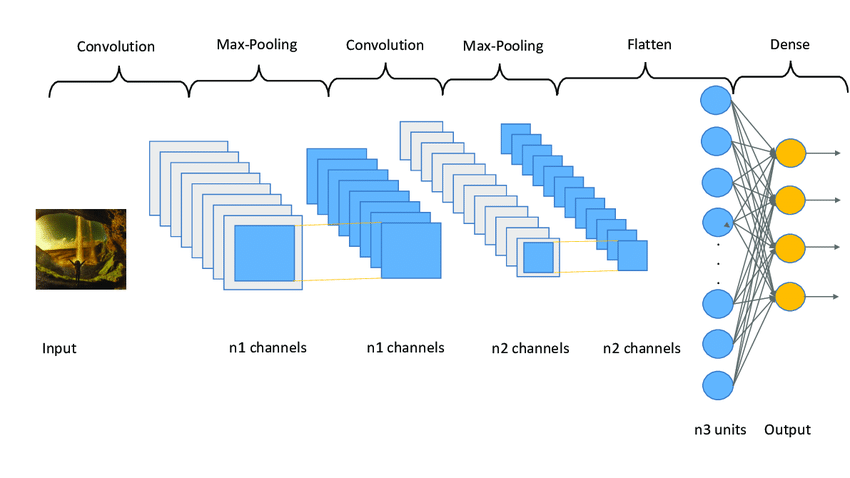
Integration with Simulation
Generative AI integrates with traditional CAD and CAE software, providing engineers with a range of feasible design options that meet predefined constraints.
The process involves setting design goals and constraints. Users leverage AI to generate numerous variations algorithmically in minutes rather than hours or days. This saves time and allows users to refine the AI-generated concepts. In the end, the measurable benefits of AI technology are accelerating design cycles, reducing prototyping costs, and enabling data-driven decision-making. This approach enhances creativity and optimizes for multi-objective criteria, leading to more efficient and innovative solutions.

How Generative AI Differs from Traditional Design Tools
Following the evolution of technology, design has significantly transformed. It started as a manual, craft-driven process. User experiences were hand-drawn sketches and physical prototypes, with trial-and-error approaches. This was labor-intensive and time-consuming, often leading to long product development cycles.
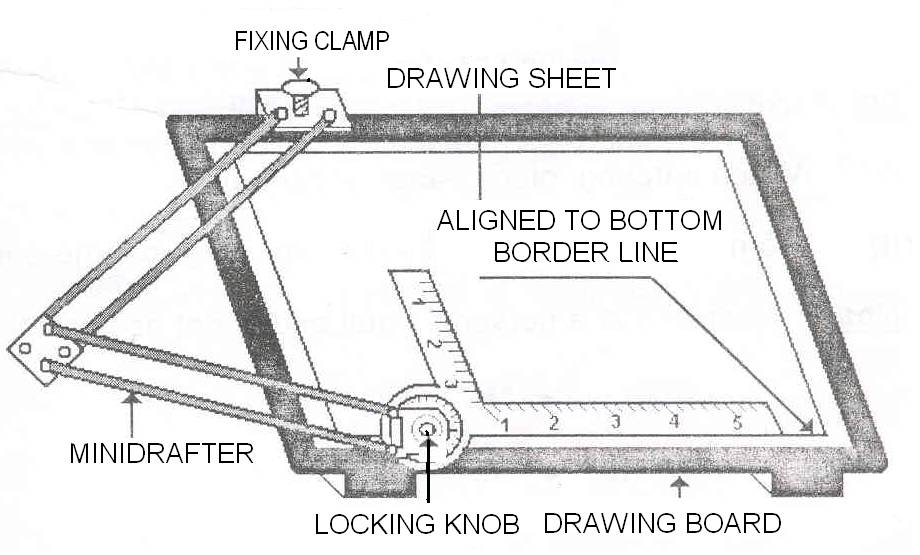
The Digital Revolution
The advent of Computer-Aided Design (CAD) in the 1960s was a game changer in the field, introducing digital tools that brought at least five advantages in design thinking:
- more detailed geometrical modeling
- faster iterations between designs
- better user experiences with more straightforward design modifications
- better and less expensive storage of information
- better sharing of information via digital formats (PDM, PLM) for users and product managers
CAD enabled design engineers to create complex geometries and visualize products in 2D and 3D. This improved efficiency and promoted innovative design thinking. However, traditional CAD still required significant manual human input, where engineers explicitly needed to define the geometry, dimensions, and constraints. This leads to talk about designs generated during the creative process.
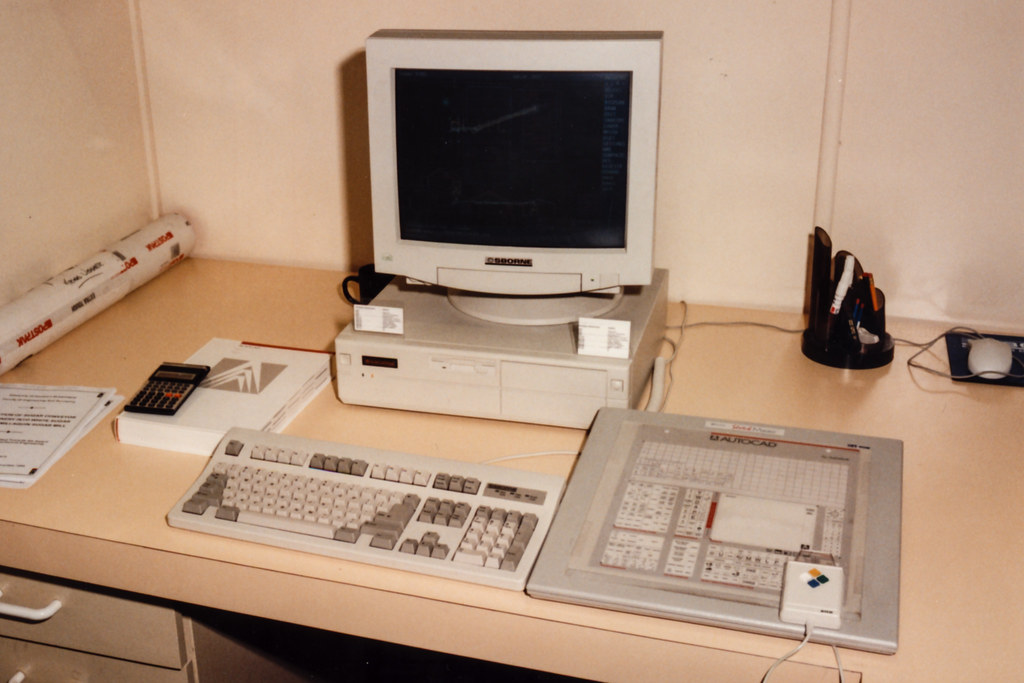
Traditional Design Tools: Manual Iteration and Optimization
Conventional design workflows follow a linear, iterative process. Engineers start with a creative concept, iteratively refined based on performance analyses. Users often deploy Computer-Aided Engineering (CAE) tools for simulations (e.g., stress analysis, fluid mechanics). The process involves manual adjustments and multiple cycles, requiring hardware resources and domain expertise in at least two fields: using the software and optimizing the design. This technology, while effective, was limited by available users (lack of democratization), human creativity, and time constraints.
This leads to exploring only a tiny portion of the design space.
Software 2.0 - Enter Generative AI: Automated Design Exploration
Generative AI represents a tremendous evolution in design. Generative AI exploits deep learning and optimization techniques to automate the exploration of design possibilities. Unlike CAD tools, which rely on manual inputs, generative AI automatically uses algorithms to generate various design solutions. The critical difference lies in how generative AI explores design spaces. Instead of starting with a fixed design and iterating on it, generative AI begins with performance objectives and constraints (e.g., weight reduction, structural strength, thermal efficiency).
AI generates and evaluates thousands of design variations using deep learning models, such as neural networks, identifying optimal solutions that human designers may not have considered.
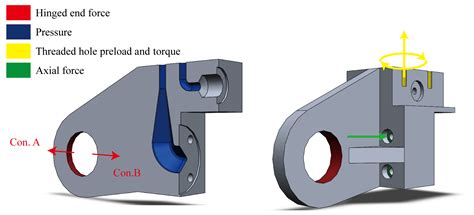
For example, topology optimization is a technique that removes non-essential material to achieve lightweight yet robust structures. This can be an impactful application of generative AI, where weight reduction is essential. Less weight can mean more performance and fuel efficiency.
The steps in generative AI for topology optimization are:
1. Input Specification: Define the design space, load conditions, and constraints (e.g., maximum stress, material usage).
2. Training Surrogate Models: Use AI predictive models trained on simulation data to predict design performance.
3. Iterative Design Generation: AI generates designs, tests them, and refines geometry to improve objectives like minimizing weight or maximizing stiffness.
4. Real-Time Feedback: Designers modify inputs interactively, and AI recalculates optimal solutions
Is AI Eating the World?
Generative AI complements traditional CAD and CAE instruments. It integrates into existing workflows and provides engineers with optimized design ideas that humans can refine further. This integration makes it possible to implement simulation-driven design, where AI-generated concepts are validated against real-world constraints, prototyped with 3D printers, and tested in the lab.

Benefits of Generative AI in Product Design
Generative AI democratizes product design by enabling faster, more efficient, and innovative approaches previously unimaginable by users of traditional methods. Below are some of the key benefits for users and corporations.
Enhancing Creativity and Innovation
We all like to expand the boundaries of our inventiveness. We want to explore innovative shapes and structures that are difficult or impossible to conceive through manual design methods. Generative AI empowers users to do this. Generative AI helps teams swiftly grasp project context and generate new product proposals.
This AI technology helps discover novel design solutions and new possibilities in aesthetics and functionality. Neural Concept enhances this creative exploration process by leveraging AI-driven design iterations, assisting engineers to uncover innovative solutions quickly. This leads us to the next advantage.
Speeding Up Design Iterations
One of generative AI’s significant advantages is reducing time for design iterations. Instead of manually tweaking designs and running simulations, generative AI automates this process, accelerating the product development cycle. Neural Concept’s platform automates design and simulations, enabling users (or algorithms controlled by users) to evaluate multiple design variations with a speeded-up time to market.
Optimizing for Performance and Cost
Generative AI optimizes designs for various factors, such as material usage and structural strength, in an optimization “loop” (see figure). AI can identify solutions that balance the required performance targets with cost-effectiveness by analyzing various design alternatives in a generative AI optimization approach that generates new shapes. Neural Concept’s platform demonstrated its capability to achieve optimized designs, creating lighter, more robust, and more cost-effective products in the automotive and aerospace industries.

Challenges for Generative AI in Product Design
Implementing generative AI in product design offers transformative potential, yet it also presents several challenges that must be addressed to leverage its capabilities thoroughly. These challenges span data requirements, integration into existing workflows, and the interpretability of AI-generated outputs. Here’s a closer look at each of these areas:
Data Requirements and Quality Concerns
Generative AI models depend on the quality of the data they are trained on. Poor data quality can lead to suboptimal designs, undermining AI’s effectiveness. So, we should focus on clean, high-quality datasets to ensure reliable outputs. Tools like Neural Concept help by leveraging industry-specific datasets and high-quality design data to train models, leading to accurate and practical design solutions.
Integration with Existing Design Workflows
Integrating generative AI into traditional CAD-based design workflows is a challenge. Many design teams are accustomed to established processes, making adopting new AI-driven solutions potentially disruptive. Neural Concept’s platform addresses this challenge and bridges generative AI models with conventional design software, ensuring efficiency without overhauling existing workflows.
Interpretability of AI-Generated Designs
A hurdle in generative AI is understanding the rationale behind AI-generated designs. Users often perceive Generative AI as a “black box,” leading to a lack of trust. A lack of interpretability and trust can lead to hesitation in adopting AI-generated solutions. Neural Concept offers simulation insights that give engineers a deeper understanding of AI-generated designs and build confidence in AI’s design recommendations.
Unlock the Power of Generative AI in Design with Deep Learning
Neural Concept uses data-driven Deep Learning models to complement traditional CFD and FEA solvers. The surrogates from Neural Concept’s platform drastically reduce simulation times with real-time responses. This empowers users to efficiently optimize aerodynamic, thermal, and structural properties with interactive design space exploration.
How Neural Concept Empowers Generative AI Design
A core strength at Neural Concept is the simulation-driven design approach. Deep learning models accelerate simulations typically relying on computational fluid dynamics (CFD) and FEA. This enables users to evaluate aerodynamic, thermal, and structural properties faster, which is critical for the automotive, aerospace, and consumer electronics industries.
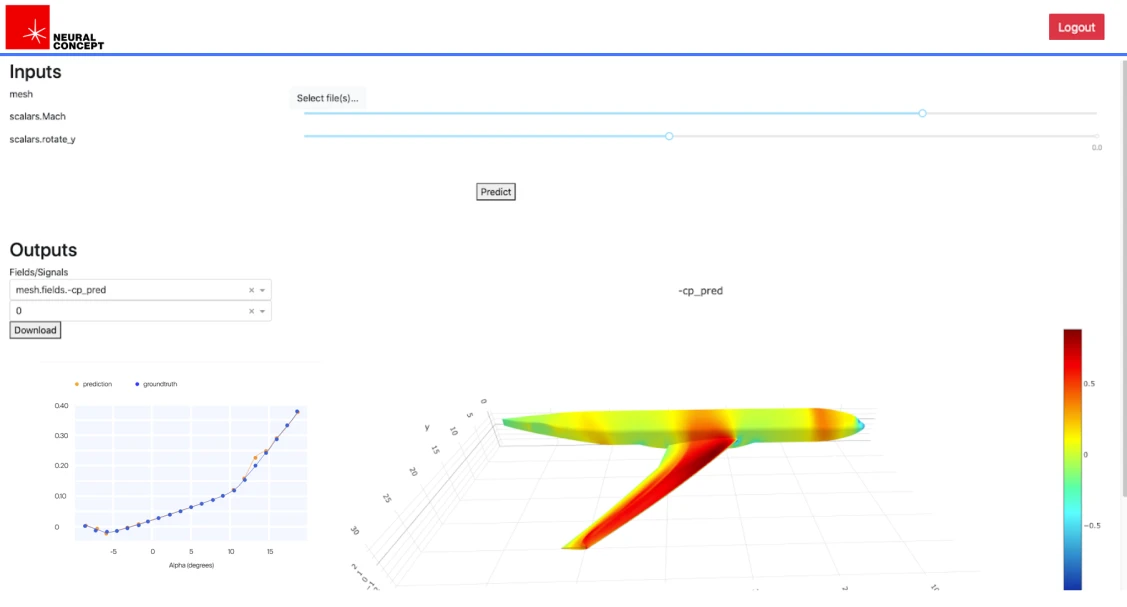
Accelerating CFD and FEA. Neural Concept’s AI-based surrogate models can predict the performance of new designs without rerunning full simulations, reducing computation times from hours or days to minutes.
Interactive Design Exploration. Users can tweak parameters like shape, size, or boundary conditions and instantly see how those changes affect the performance. This interactivity supports a more iterative, exploratory design approach, enabling teams to converge to optimal solutions much faster.
Future Trends in Generative AI for Product Design
We will explore future product design trends in generative AI for design. They include real-time generative design, enabling instant exploration of optimized solutions, and AI-driven digital twins that simulate real-world performance for better decision-making. Adaptive AI also transforms workflows by learning and evolving with user needs. These innovations accelerate development, improve precision, and support customized designs across every industry.

AI-Driven Digital Twins
AI-driven digital twins are virtual models that use AI and machine learning to simulate the physical behavior of systems in real-time. These models integrate sensor data, simulation algorithms, and predictive analytics to monitor and predict system performance. Continuous updates based on live data enhance accuracy in failure prediction and operational efficiency.
Adaptive AI systems
Adaptive AI systems dynamically adjust their behavior based on changing data and environments without explicit reprogramming. Leveraging techniques like reinforcement learning, online learning, and meta-learning, these systems evolve to optimize performance in real-time. They are used in applications requiring personalization, anomaly detection, and continuous improvement, such as autonomous vehicles, predictive maintenance, and customer interaction platforms. Their ability to learn from experience enhances flexibility and resilience in complex, dynamic tasks.
Case Studies: Success Stories with Neural Concept Shape
The following success stories showcase real-world examples of companies using Neural Concept’s platform for generative AI in product design. The specific use cases include automotive, aerospace, and industrial design examples, demonstrating the tangible benefits of using the Neural Concept technology in engineering performance improvement.
Subaru Success Story - Automotive Industry
Subaru applied Neural Concept technology to optimize the die face shape design in their production engineering, specifically for press molding analysis. Analyzing metal forming in stamping operations using CAE software took up to 48 hours, slowing the development process of new vehicle models. Neural Concept’s deep learning capabilities reduced the time to just 2 minutes, accurately predicting material thickness reduction. This speeds up the Subaru design cycle, supporting their goal to launch next-generation vehicles while improving production efficiency and reducing time-to-market.
Danfoss Success Story - Industrial Design
Danfoss partnered with Neural Concept to optimize check valve design using Neural Concept’s technology. Neural Concept’s platform evaluated these parameters, reducing design iteration time from hours to seconds. Danfoss conducted multi-objective optimization, assessing 750 designs over only 10 hours! This led to a 10% improvement in mass flow rate at full valve opening and explored a broad design space, enhancing efficiency and performance.

Airbus Success Story - Aerospace Industry
Neural Concept and industry leader Airbus are collaborating to accelerate engineering processes in fluid dynamics, structural engineering, and electromagnetics. The initial focus was Computational Fluid Dynamics development, where Airbus engineers successfully tested Neural Concept’s platform on external aerodynamics. In 2019, Neural Concept and Airbus showcased real-time CFD simulations using 3D Convolutional Neural Networks. This breakthrough enabled predictions in 30 milliseconds; this speed is compared to minutes or even days with traditional simulation software. s. This collaboration within the AI platform showed how 3D deep learning can revolutionize the aerospace industry by creating innovative design solutions.
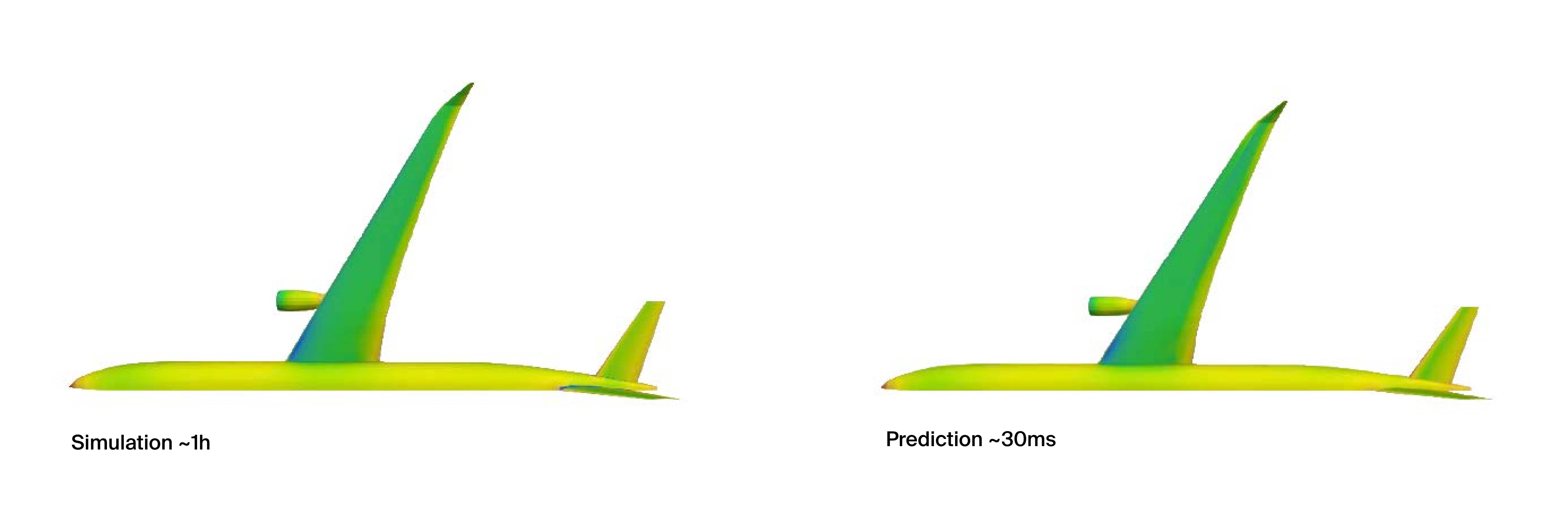
The Role of Generative AI in Product Design and Development
What is generative AI in design, and how does it enhance product development?
Generative AI uses algorithms to generate new content based on predefined parameters. This could mean developing new design concepts or optimizing existing ones in product design. Product designers using generative AI can explore various possibilities without manually creating variations. This speeds up the product design process and provides designers with insights that might not have been discovered through traditional methods. Integrating artificial intelligence into product design workflows has opened up new possibilities for innovation, allowing designers to create faster, more efficient, and more user-focused products.
At the heart of generative AI’s impact on product design are:
- Analyzing large datasets: By leveraging big data, AI can help designers make data-driven decisions that align with user needs and preferences.
- Suggesting innovative solutions: The models, built on training data, can suggest designs that meet specific criteria such as durability, cost-effectiveness and manufacturability. This shift from traditional design methods to AI-powered design systems enables product designers to be more creative and efficient.
Generative AI Deployment
Generative AI in product design is particularly useful in the early stages of innovation, where new ideas are formulated and prototypes are created. AI can rapidly generate multiple iterations of a product design based on user prompts or input parameters. This process leads to faster experimentation and decision-making, speeding up the development cycle.
The Benefits of Generative AI in Product Development
Business: One of the most notable advantages of generative AI in product development is time and reduced cost savings. Product designers and engineers can focus on higher-level strategic decisions by automating time-consuming tasks such as prototyping, testing, and generating design variations. Additionally, AI can identify inefficiencies and suggest improvements in design, material usage, and manufacturing processes.
Creativity: AI tools can integrate user research and feedback, such as user behavior and sentiment analysis, for real-time adjustment. This ensures the final product is innovative and aligned with market demands, customers, and user expectations.
Generative AI also impacts rapid prototyping and 3D printing. Physical prototypes can be costly and time-consuming. AI allows designers to create and test digital prototypes at a fraction of the cost and time. This means more iterations and quicker adjustments, essential in fast-paced industries where time-to-market is critical.
How AI Tools Are Changing Product Design
Integrating AI into product design has created a “paradigm shift” in product creation. Designers now have access to sophisticated AI software that can assist in virtually every aspect of the design process, from user research to concept generation to final production.
These AI softwares are not meant to replace human input for product designers but to augment their capabilities with user interaction, providing engineers with powerful insights and suggestions that can lead to better decision-making. In particular, product designers use generative AI to work faster and more efficiently. AI can analyze vast amounts of data, such as user feedback, product performance, and design trends, to suggest improvements or alternatives. This data-driven approach helps ensure innovative new product designs align with user needs and market demands.
For instance, AI can analyze user feedback from interactions with prototypes to determine which design elements resonate most with users. This user feedback is then integrated into the next iteration of the design, creating a feedback loop that results in better products over time. This iterative process of refinement, driven by AI, enhances the product design process and ensures that the final product meets user expectations and stands out in the marketplace.
Furthermore, generative AI can process user feedback more effectively with the increased capability to understand natural language. This makes it easier for tasks to be refined and optimized based on iterative user feedback.
AI in the Innovation Process
AI’s role in innovation and creation in industry is undeniable. AI capabilities augment inventiveness that relies solely on human intuition and expertise. Analyzing big datasets and generating new proposals, AI can help designers identify new opportunities for innovation, sometimes out of the human “bias” or because traditional methods are too slow in response—imagine waiting 8 hours to get an aerodynamic prediction following a design change! AI has proven to be a game changer in industries ranging from automotive to consumer electronics, where the speed and efficiency of design have become critical competitive factors. Product designers use AI to explore new design possibilities, simulate different product variations, and forecast a product’s market performance. This data-driven approach ensures that designers reduce the risk of expensive mistakes. Furthermore, AI’s ability to simulate user experiences and predict user behavior allows designer engineers to create products more aligned with user needs and preferences.
Example of Generative AI in Product Development
A practical instance of generative AI in product development can be seen in the automotive industry, where car manufacturers use AI to design more efficient and innovative vehicle parts. Generative AI models can create optimized designs for chassis or interior elements, considering factors like material strength, weight, and aerodynamics. These AI-generated designs are then tested virtually, with AI predicting their performance under various conditions.
By using Generative AI to generate a wide range of design concepts based on user preferences and market trends, companies in consumer electronics can quickly develop prototypes and bring new products to market faster. AI-driven design processes in these industries help companies stay ahead of the competition by rapidly adapting to changing consumer demands.

Could Can AI Replace Product Design?
Despite the many advancements in generative AI, the role of human designers remains crucial. AI tools are powerful, but they lack the creativity, intuition, and deep understanding of human needs that product designers bring. Instead, AI should be viewed as a complement to human creativity, providing designers with powerful tools that help them work faster, refine their ideas, and create more innovative services and products.
The Future of Generative AI Product Design. Conclusion
AI technology evolves, and its role in product design will only become more significant. The capabilities of AI systems are expanding, allowing for even greater integration of AI into the design process. AI has become more sophisticated. Platforms such as Neural Concept can provide more personalized design solutions and adapt to different contexts; this explains why many organizations use generative AI in product and service development. As AI adoption grows, collaboration between AI systems and designers will become tighter. Product designers are helped out of repetitive tasks and focus on higher-level challenges. This evolution will create more innovative products that better meet users’ needs while improving efficiency and reducing costs.
In conclusion, generative AI is an indispensable tool in product design. Generative AI allows product teams to leverage data to reduce costs in product development. AI automates routine and repetitive tasks, generating new ideas and optimizing the design process. AI empowers designers to be more creative, efficient, and data-driven. While AI cannot replace human designers, it is a powerful assistant, helping them create products that meet the market’s ever-changing demands. The collaboration between humans and artificial intelligence will shape future product design.