Design Optimization of Heat Exchangers

Heat exchangers are widely used in a large range of industries and applications, for example in the automotive industry for HVAC systems, with the aim to control the degree of comfort for driver and passengers in the vehicle. In the quest for a greener mobility and more and more ambitious regulations, heat exchanger systems need to be as efficient as possible. Indeed, electric vehicles (EV) have a lower-energy storage capacity, and heat exchangers may consume a substantial amount of the total energy stored, considerably reducing the vehicle range, which is one of the most important parameters for EV acceptability. Hence, even a minor improvement in the overall performance of the unit can be a game-changer for the manufacturer, as well as the final customer.
There is a wide range of acceptable designs for heat exchangers, and the number of parameters describing the heat exchanger geometry can quickly rise as the design becomes more and more complex. Since the corresponding numerical simulation is computationally very expensive, the design engineer can only afford to iterate on a few design parameters to try and improve the system.
We built a surrogate model allowing to predict in real-time the performance of various heat exchanger designs, with different topologies.
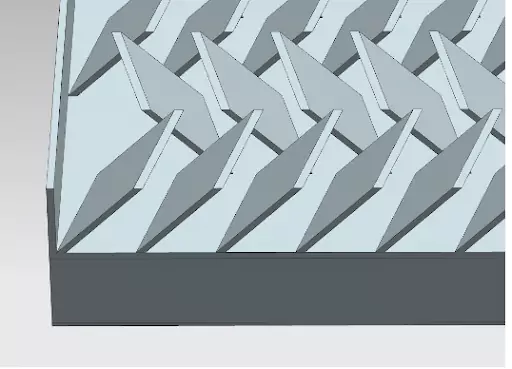
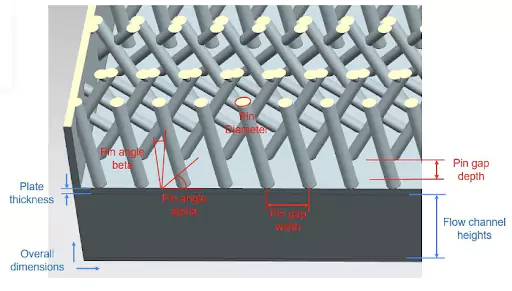
The surrogate model is able to predict very accurately the overall efficiency of the system, as well as temperature and pressure drops at the outlets. The engineer is now able to interact in real-time with the heat exchanger designs, to iterate efficiently between different geometries and topologies.

Moreover, the model is also predicting the flow within the volume, providing engineers with a better intuitive understanding of the phenomenon. On top of the surrogate model, the optimization library of Neural Concept was used, bringing substantial improvements to the final design.
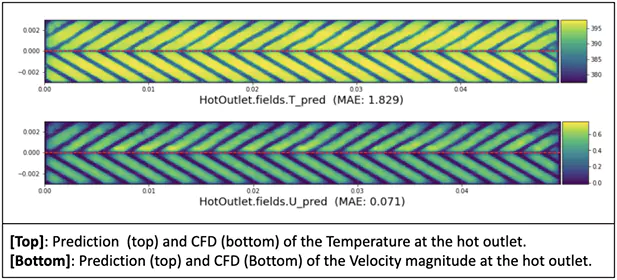


















































