Transonic Airfoil Flow Design Evaluation With Deep Learning Models
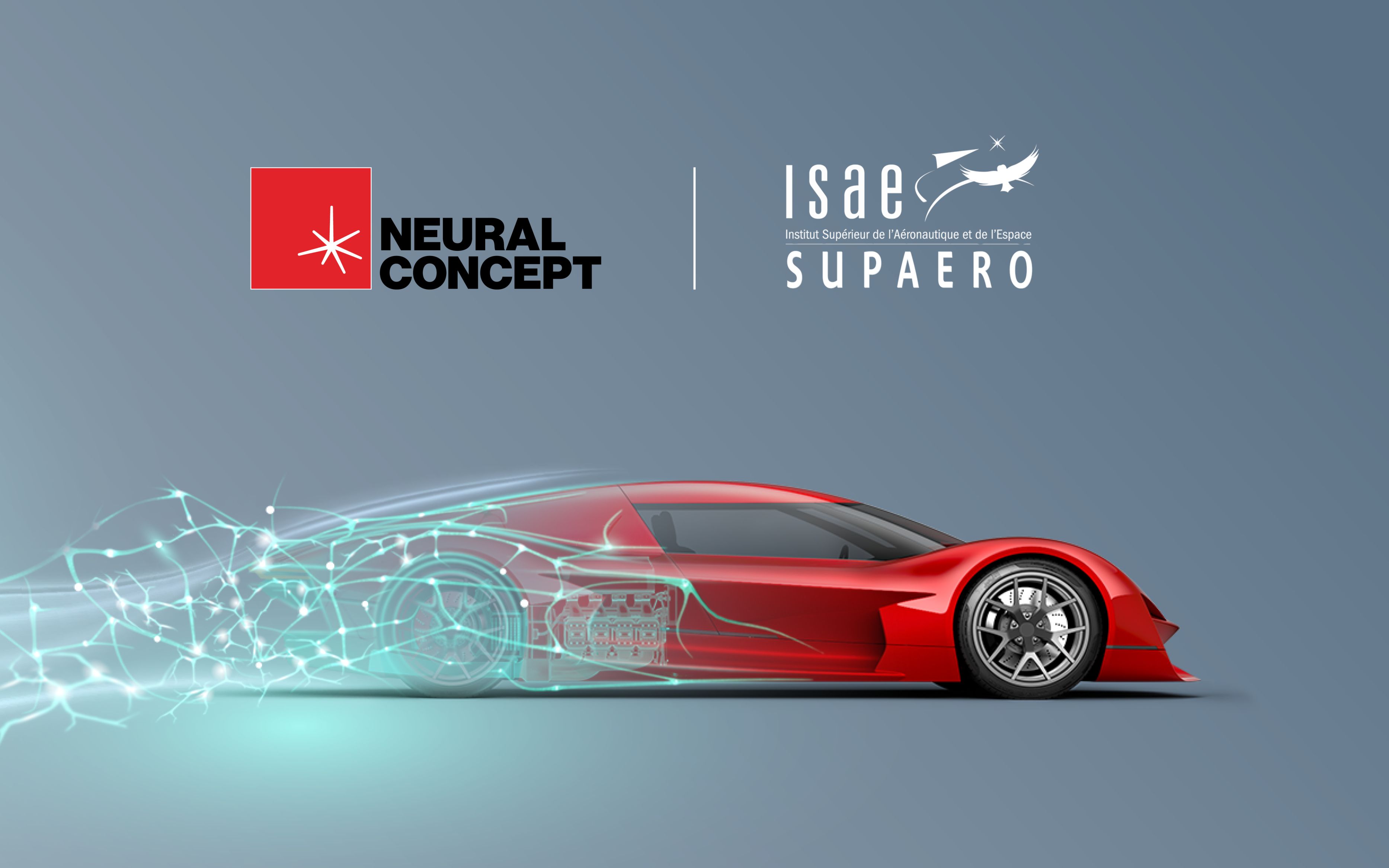
ISAE-Supaero, a world leader in aerospace engineering higher education in France, and Neural Concept, have developed a new approach to high Mach aircraft design. From an incompressible flow simulation, Neural Concept’s Neural Networks are able to predict flow simulations at a high Mach number. Therefore, a full description of the simulations for the flight envelope (Mach-Alpha), at high Mach, can be obtained accurately by running a single inexpensive simulation.
This methodology has been integrated into NCS and deployed into a NCS/Lite app, currently at demo stage.
High-Mach simulations are expensive and necessary for Aircraft design
For low-speed regimes, the flow over an airfoil can be considered as incompressible, thus simplifying the underlying physics, and making it simpler to study, analyze and simulate. However, when reaching higher speeds, the flow enters a transonic regime. Therefore, the simplifying assumption is not valid anymore as compressibility effects start to influence the overall performance of the airfoil, such as the formation of shock waves. The physics underlying these phenomena become then much more challenging to model.

Hence, it is critical for the engineers to be able to understand the influence of these compressibility effects, at various operating conditions (flow velocity, angle of attack) to ensure reliability and uniformity in the performances of the design. To do so, it is normally necessary for Aerospace engineers to run many CFD simulations for various boundary conditions to cover the whole space of operating conditions. For transonic flows, with highly non-linear behavior, these simulations are very time-consuming and expensive, creating a bottleneck in the evaluation process.
Deep-Learning can predict many high-mach simulations from a single, non-expensive one

The proposed methodology is based on Neural Concept’s Deep Learning models, which are trained to “transpose” simulations from low-mach to high-mach. It makes it possible to predict the whole Mach envelope, i.e., pressure fields at the various angle of attack and higher Mach numbers in a few seconds. It uses as input a computationally non-expensive single incompressible flow simulation.
In this setting, the user is able to upload an airfoil geometry, with the corresponding simulation at a low Mach number. The model then predicts the corresponding pressure profile at a higher Mach number which can be specified by the user. This allows for much faster iterations between designs, and a better understanding of the complex phenomena occurring above a given speed.
The results presented in the report show that Neural Concept’s Deep Learning-based models can accurately describe the highly non-linear regions on the airfoil, where large pressure gradients occur, making it a reliable and robust solution to model compressibility effects of transonic flows on airfoils.
This approach has proven more accurate than the standard methodology used by Neural Concept’s users, where only a geometry would be fed into the predictor. Adding here the output of an incompressible flow simulation as an input to the Neural Network, seems to improve its generalization power. This is easily done in NCS by exploiting the options to provide so-called Input-Fields to the Neural Network.
For more information, please read the abstract:
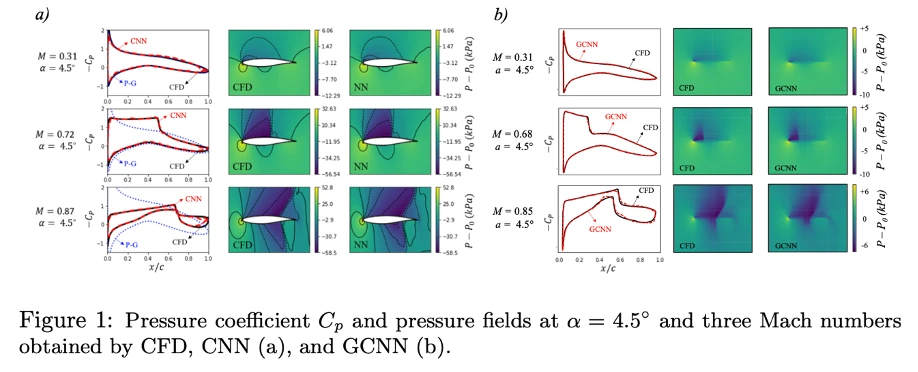
© ISAE-Supaero: M.Bauerheim, D.Costero, V. Chapin, N.Gourdain & Neural Concept : L.Zampieri, P.Baqué